Data Mining Han And Kamber Solution Pdf
Jiawei Han, Micheline Kamber and Jian Pei. Data Mining: Concepts and Techniques, 3rd ed. The Morgan Kaufmann Series in Data Management Systems Morgan Kaufmann Publishers, July 2011.
. Dedication. Foreword. Foreword to Second Edition. Preface. Organization of the Book. To the Instructor.
To the Student. To the Professional. Book Web Sites with Resources. Acknowledgments. Third Edition of the Book.
Second Edition of the Book. First Edition of the Book. About the Authors. 1. Introduction. Publisher Summary.
1.1 Why Data Mining?. 1.2 What Is Data Mining?. 1.3 What Kinds of Data Can Be Mined?. 1.4 What Kinds of Patterns Can Be Mined?. 1.5 Which Technologies Are Used?. 1.6 Which Kinds of Applications Are Targeted?.
1.7 Major Issues in Data Mining. 1.8 Summary. 1.9 Exercises. 1.10 Bibliographic Notes. 2. Getting to Know Your Data. Publisher Summary.
2.1 Data Objects and Attribute Types. 2.2 Basic Statistical Descriptions of Data. 2.3 Data Visualization.
2.4 Measuring Data Similarity and Dissimilarity. 2.5 Summary. 2.6 Exercises. 2.7 Bibliographic Notes.
3. Data Preprocessing. Publisher Summary. 3.1 Data Preprocessing: An Overview. 3.2 Data Cleaning.
3.3 Data Integration. 3.4 Data Reduction. 3.5 Data Transformation and Data Discretization. 3.6 Summary. 3.7 Exercises.
3.8 Bibliographic Notes. 4. Data Warehousing and Online Analytical Processing. Publisher Summary. 4.1 Data Warehouse: Basic Concepts. 4.2 Data Warehouse Modeling: Data Cube and OLAP. 4.3 Data Warehouse Design and Usage.
4.4 Data Warehouse Implementation. 4.5 Data Generalization by Attribute-Oriented Induction. 4.6 Summary. 4.7 Exercises. Bibliographic Notes. 5.
Data Cube Technology. Publisher Summary. 5.1 Data Cube Computation: Preliminary Concepts. 5.2 Data Cube Computation Methods. 5.3 Processing Advanced Kinds of Queries by Exploring Cube Technology. 5.4 Multidimensional Data Analysis in Cube Space. 5.5 Summary.
5.6 Exercises. 5.7 Bibliographic Notes. 6.
Mining Frequent Patterns, Associations, and Correlations: Basic Concepts and Methods. Publisher Summary. 6.1 Basic Concepts. 6.2 Frequent Itemset Mining Methods. 6.3 Which Patterns Are Interesting?—Pattern Evaluation Methods. 6.4 Summary.
6.5 Exercises. 6.6 Bibliographic Notes. 7. Advanced Pattern Mining. Publisher Summary. 7.1 Pattern Mining: A Road Map.
7.2 Pattern Mining in Multilevel, Multidimensional Space. 7.3 Constraint-Based Frequent Pattern Mining. 7.4 Mining High-Dimensional Data and Colossal Patterns.
7.5 Mining Compressed or Approximate Patterns. 7.6 Pattern Exploration and Application. 7.7 Summary. 7.8 Exercises. 7.9 Bibliographic Notes. 8. Classification: Basic Concepts.
Publisher Summary. 8.1 Basic Concepts.
8.2 Decision Tree Induction. 8.3 Bayes Classification Methods. 8.4 Rule-Based Classification. 8.5 Model Evaluation and Selection. 8.6 Techniques to Improve Classification Accuracy.
8.7 Summary. 8.8 Exercises. 8.9 Bibliographic Notes. 9. Classification: Advanced Methods. Publisher Summary. 9.1 Bayesian Belief Networks.
9.2 Classification by Backpropagation. 9.3 Support Vector Machines. 9.4 Classification Using Frequent Patterns. 9.5 Lazy Learners (or Learning from Your Neighbors). 9.6 Other Classification Methods. 9.7 Additional Topics Regarding Classification. Summary.
9.9 Exercises. 9.10 Bibliographic Notes. 10.
Cluster Analysis: Basic Concepts and Methods. Publisher Summary. 10.1 Cluster Analysis. 10.2 Partitioning Methods. 10.3 Hierarchical Methods.
10.4 Density-Based Methods. 10.5 Grid-Based Methods. 10.6 Evaluation of Clustering. 10.7 Summary. 10.8 Exercises. Free habbo accounts.
10.9 Bibliographic Notes. 11.
Han And Kamber
Advanced Cluster Analysis. Publisher Summary. 11.1 Probabilistic Model-Based Clustering. 11.2 Clustering High-Dimensional Data. 11.3 Clustering Graph and Network Data.
11.4 Clustering with Constraints. Summary. 11.6 Exercises.
11.7 Bibliographic Notes. 12. Outlier Detection. Publisher Summary. 12.1 Outliers and Outlier Analysis.
12.2 Outlier Detection Methods. 12.3 Statistical Approaches. 12.4 Proximity-Based Approaches. 12.5 Clustering-Based Approaches.
12.6 Classification-Based Approaches. 12.7 Mining Contextual and Collective Outliers. 12.8 Outlier Detection in High-Dimensional Data. 12.9 Summary. 12.10 Exercises.
12.11 Bibliographic Notes. 13. Data Mining Trends and Research Frontiers.
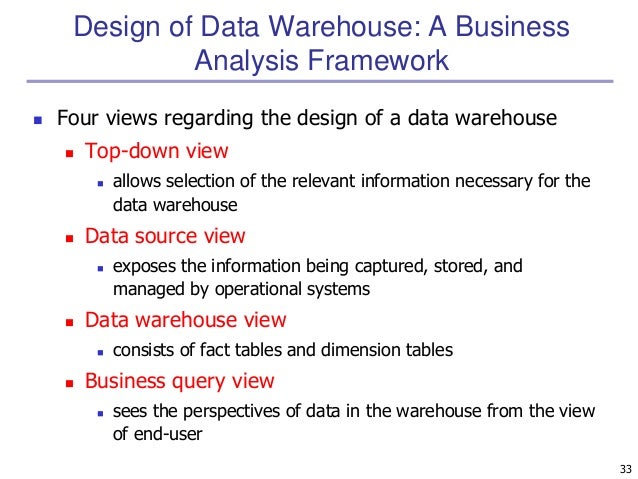
Publisher Summary. 13.1 Mining Complex Data Types. 13.2 Other Methodologies of Data Mining. 13.3 Data Mining Applications. 13.4 Data Mining and Society. 13.5 Data Mining Trends. 13.6 Summary.
13.7 Exercises. 13.8 Bibliographic Notes. Bibliography.
Index. Data Mining: Concepts and Techniques provides the concepts and techniques in processing gathered data or information, which will be used in various applications. Specifically, it explains data mining and the tools used in discovering knowledge from the collected data. This book is referred as the knowledge discovery from data (KDD). It focuses on the feasibility, usefulness, effectiveness, and scalability of techniques of large data sets. After describing data mining, this edition explains the methods of knowing, preprocessing, processing, and warehousing data.
It then presents information about data warehouses, online analytical processing (OLAP), and data cube technology. Then, the methods involved in mining frequent patterns, associations, and correlations for large data sets are described. The book details the methods for data classification and introduces the concepts and methods for data clustering.
The remaining chapters discuss the outlier detection and the trends, applications, and research frontiers in data mining. This book is intended for Computer Science students, application developers, business professionals, and researchers who seek information on data mining. Key Features. 'A well-written textbook (2nd ed., 2006; 1st ed., 2001) on data mining or knowledge discovery. The text is supported by a strong outline. The authors preserve much of the introductory material, but add the latest techniques and developments in data mining, thus making this a comprehensive resource for both beginners and practitioners.
The focus is data—all aspects. The presentation is broad, encyclopedic, and comprehensive, with ample references for interested readers to pursue in-depth research on any technique. Summing Up: Highly recommended. Upper-division undergraduates through professionals/practitioners.'
-CHOICE 'This interesting and comprehensive introduction to data mining emphasizes the interest in multidimensional data mining-the integration of online analytical processing (OLAP) and data mining. Some chapters cover basic methods, and others focus on advanced techniques. The structure, along with the didactic presentation, makes the book suitable for both beginners and specialized readers.' -ACM’s Computing Reviews.com 'We are living in the data deluge age. The Data Mining: Concepts and Techniques shows us how to find useful knowledge in all that data.
Data Mining Han Pdf
Thise 3rd editionThird Edition significantly expands the core chapters on data preprocessing, frequent pattern mining, classification, and clustering. The bookIt also comprehensively covers OLAP and outlier detection, and examines mining networks, complex data types, and important application areas. The book, with its companion website, would make a great textbook for analytics, data mining, and knowledge discovery courses.' -Gregory Piatetsky, President, KDnuggets 'Jiawei, Micheline, and Jian give an encyclopaedic coverage of all the related methods, from the classic topics of clustering and classification, to database methods (association rules, data cubes) to more recent and advanced topics (SVD/PCA, wavelets, support vector machines). Overall, it is an excellent book on classic and modern data mining methods alike, and it is ideal not only for teaching, but as a reference book.'
Solution Of Data Mining By Han And Kamber 3rd Edition Pdf
-From the foreword by Christos Faloutsos, Carnegie Mellon University 'A very good textbook on data mining, this third edition reflects the changes that are occurring in the data mining field. It adds cited material from about 2006, a new section on visualization, and pattern mining with the more recent cluster methods.

It’s a well-written text, with all of the supporting materials an instructor is likely to want, including Web material support, extensive problem sets, and solution manuals. Though it serves as a data mining text, readers with little experience in the area will find it readable and enlightening. That being said, readers are expected to have some coding experience, as well as database design and statistics analysis knowledgeTwo additional items are worthy of note: the text’s bibliography is an excellent reference list for mining research; and the index is very complete, which makes it easy to locate information.

Also, researchers and analysts from other disciplines-for example, epidemiologists, financial analysts, and psychometric researchers-may find the material very useful.' -Computing Reviews 'Han (engineering, U. Of Illinois-Urbana-Champaign), Micheline Kamber, and Jian Pei (both computer science, Simon Fraser U., British Columbia) present a textbook for an advanced undergraduate or beginning graduate course introducing data mining. Students should have some background in statistics, database systems, and machine learning and some experience programming. Among the topics are getting to know the data, data warehousing and online analytical processing, data cube technology, cluster analysis, detecting outliers, and trends and research frontiers. Chapter-end exercises are included.' -SciTech Book News 'This book is an extensive and detailed guide to the principal ideas, techniques and technologies of data mining.
The book is organised in 13 substantial chapters, each of which is essentially standalone, but with useful references to the book’s coverage of underlying concepts. A broad range of topics are covered, from an initial overview of the field of data mining and its fundamental concepts, to data preparation, data warehousing, OLAP, pattern discovery and data classification.
The final chapter describes the current state of data mining research and active research areas.' Jiawei Han is Professor in the Department of Computer Science at the University of Illinois at Urbana-Champaign.
Well known for his research in the areas of data mining and database systems, he has received many awards for his contributions in the field, including the 2004 ACM SIGKDD Innovations Award. He has served as Editor-in-Chief of ACM Transactions on Knowledge Discovery from Data, and on editorial boards of several journals, including IEEE Transactions on Knowledge and Data Engineering and Data Mining and Knowledge Discovery. Jian Pei is currently a Canada Research Chair (Tier 1) in Big Data Science and a Professor in the School of Computing Science at Simon Fraser University. He is also an associate member of the Department of Statistics and Actuarial Science. He is a well-known leading researcher in the general areas of data science, big data, data mining, and database systems. His expertise is on developing effective and efficient data analysis techniques for novel data intensive applications. He is recognized as a Fellow of the Association of Computing Machinery (ACM) for his “contributions to the foundation, methodology and applications of data mining” and as a Fellow of the Institute of Electrical and Electronics Engineers (IEEE) for his “contributions to data mining and knowledge discovery”.
He is the editor-in-chief of the IEEE Transactions of Knowledge and Data Engineering (TKDE), a director of the Special Interest Group on Knowledge Discovery in Data (SIGKDD) of the Association for Computing Machinery (ACM), and a general co-chair or program committee co-chair of many premier conferences.
Jiawei Han and Micheline Kamber Data Mining: Concepts and Techniques, 2 nd ed. The Morgan Kaufmann Series in Data Management Systems, Jim Gray, Series Editor, March 2006. ISBN 1-55860-901-6 “The second edition of Han and Kamber Data Mining: Concepts and Techniques updates and improves the already comprehensive coverage of the first edition and adds coverage of new and important topics, such as mining stream data, mining social networks, and mining spatial, multimedia, and other complex data. This book will be an excellent textbook for courses on Data Mining and Knowledge Discovery.” -Gregory Piatetsky-Shapiro, President, “The second edition is the most complete and up-to-date presentation on this topic.
Compared to the already comprehensive and thorough coverage of the first edition, it adds the state-of-the-art research results in new topics such as mining stream, time-series and sequence data as well as mining spatial, multimedia, text and Web data. This book is a must-have for all instructors, researchers, developers and users in the area of data mining and knowledge discovery.” - Hans-Peter Kriegel, University of Munich, Germany.